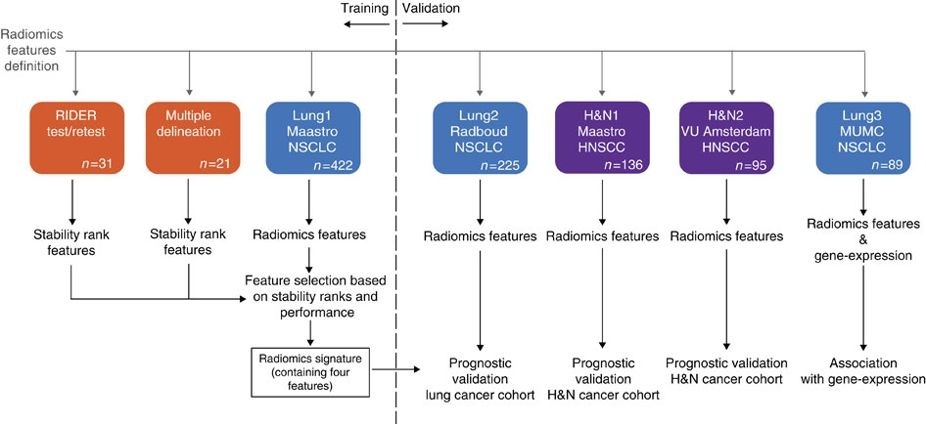
RIDER-LungCT-Seg | RIDER Lung CT Segmentation Labels from: Decoding tumour phenotype by noninvasive imaging using a quantitative radiomics approach
DOI: 10.7937/tcia.2020.jit9grk8 | Data Citation Required | Analysis Result
Location | Subjects | Size | Updated | |||
---|---|---|---|---|---|---|
Lung | Chest | 31 | Tumor segmentations | 2021/10/28 |
Summary
This dataset contains images from 31 out of the 32 non-small cell lung cancer (NSCLC) patients in the RIDER Lung CT collection on TCIA. For these subjects a radiation oncologist was blinded to the all delineations of the 3D volume of the gross tumor volume. They were then asked to manually delineate the gross tumour volume in both the test image and the re-test image. The process was repeated using an in-house autosegmentation method. There is no clinical outcome data associated with this dataset.
This dataset refers to the RIDER dataset of the study published in Nature Communications (http://doi.org/10.1038/ncomms5006). In short, this publication used the dataset to select for repeatable radiomics features in a test-retest context. Radiomics refers to the comprehensive quantification of tumour phenotypes by applying a large number of quantitative image features. In the published analysis, 440 features quantifying tumour image intensity, shape and texture, were extracted. We found that a large number of radiomic features have prognostic power in independent data sets, many of which were not identified as significant before. Radiogenomics analysis revealed that a prognostic radiomic signature, capturing intra-tumour heterogeneity, was associated with underlying gene-expression patterns. These data suggest that radiomics identifies a general prognostic phenotype existing in both lung and head-and-neck cancer. This may have a clinical impact as imaging is routinely used in clinical practice, providing an unprecedented opportunity to improve decision-support in cancer treatment at low cost.
Other data sets in the Cancer Imaging Archive that were used in the same study published in Nature Communications: NSCLC-Radiomics, NSCLC-Radiomics-Genomics, NSCLC-Radiomics-Interobserver1, HEAD-NECK-RADIOMICS-HN1.
Data Access
Version 2: Updated 2021/10/28
The authors of this dataset agreed to change the license to permit commercial use. The actual dataset remains unchanged.
Title | Data Type | Format | Access Points | Subjects | License | |||
---|---|---|---|---|---|---|---|---|
Gross Tumor Volume Segmentation - | SEG, RTSTRUCT | DICOM | Download requires NBIA Data Retriever |
31 | 43 | 118 | 118 | CC BY 3.0 |
Collections Used In This Analysis Result
Title | Data Type | Format | Access Points | Subjects | License | |||
---|---|---|---|---|---|---|---|---|
Corresponding Original CT Images from RIDER Lung CT - | CT | DICOM | Requires NBIA Data Retriever |
31 | 43 | 59 | 14,319 | CC BY 3.0 |
Citations & Data Usage Policy
Data Citation Required: Users must abide by the TCIA Data Usage Policy and Restrictions. Attribution must include the following citation, including the Digital Object Identifier:
Data Citation |
|
Wee, L., Aerts, H., Kalendralis, P., & Dekker, A. (2020). RIDER Lung CT Segmentation Labels from: Decoding tumour phenotype by noninvasive imaging using a quantitative radiomics approach [Data set]. The Cancer Imaging Archive. https://doi.org/10.7937/tcia.2020.jit9grk8 |
Detailed Description
- (RIDER-2283289298) only has segmentations associated with the retest.
- (RIDER-5195703382) only has segmentations associated with the test.
- (RIDER-8509201188) only has segmentations associated with the test.
- (RIDER-9762593735) not included in the data set due to missing delineations.
Publications Using This Data
TCIA maintains a list of publications that leverage TCIA data. If you have a manuscript you’d like to add please contact the TCIA Helpdesk.
Publication Citation |
|
Aerts, H. J. W. L., Velazquez, E. R., Leijenaar, R. T. H., Parmar, C., Grossmann, P., Carvalho, S., Bussink, J., Monshouwer, R., Haibe-Kains, B., Rietveld, D., Hoebers, F., Rietbergen, M. M., Leemans, C. R., Dekker, A., Quackenbush, J., Gillies, R. J., & Lambin, P. (2014). Decoding tumour phenotype by noninvasive imaging using a quantitative radiomics approach. Nature Communications, 5(1). https://doi.org/10.1038/ncomms5006 |
TCIA Citation |
|
Clark, K., Vendt, B., Smith, K., Freymann, J., Kirby, J., Koppel, P., Moore, S., Phillips, S., Maffitt, D., Pringle, M., Tarbox, L., & Prior, F. (2013). The Cancer Imaging Archive (TCIA): Maintaining and Operating a Public Information Repository. In Journal of Digital Imaging (Vol. 26, Issue 6, pp. 1045–1057). Springer Science and Business Media LLC. https://doi.org/10.1007/s10278-013-9622-7 |