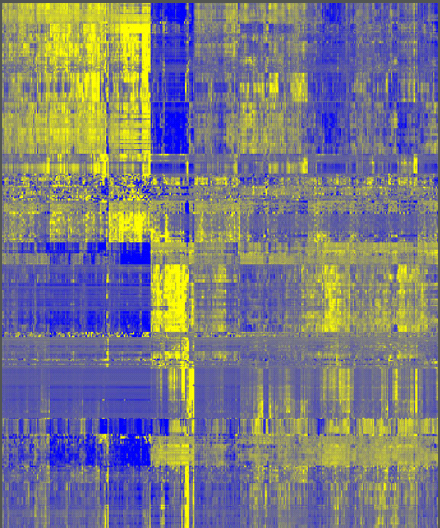
NSCLC-Radiomics-Genomics | NSCLC-Radiomics-Genomics
DOI: 10.7937/K9/TCIA.2015.L4FRET6Z | Data Citation Required | Image Collection
Location | Species | Subjects | Data Types | Cancer Types | Size | Status | Updated | |
---|---|---|---|---|---|---|---|---|
Lung | Human | 89 | CT | Lung Cancer | Clinical, Genomics | Public, Complete | 2014/07/02 |
Summary
This collection contains images from 89 non-small cell lung cancer (NSCLC) patients that were treated with surgery. For these patients pretreatment CT scans, gene expression, and clinical data are available. This dataset refers to the Lung3 dataset of the study published in Nature Communications.
In short, this publication applies a radiomic approach to computed tomography data of 1,019 patients with lung or head-and-neck cancer. Radiomics refers to the comprehensive quantification of tumour phenotypes by applying a large number of quantitative image features. In present analysis 440 features quantifying tumour image intensity, shape and texture, were extracted. We found that a large number of radiomic features have prognostic power in independent data sets, many of which were not identified as significant before. Radiogenomics analysis revealed that a prognostic radiomic signature, capturing intra-tumour heterogeneity, was associated with underlying gene-expression patterns. These data suggest that radiomics identifies a general prognostic phenotype existing in both lung and head-and-neck cancer. This may have a clinical impact as imaging is routinely used in clinical practice, providing an unprecedented opportunity to improve decision-support in cancer treatment at low cost.
The dataset described here (Lung3) was used to investigate the association of radiomic imaging features with gene-expression profiles. The Lung2 dataset used for training the radiomic biomarker and consisting of 422 NSCLC CT scans with outcome data can be found here: NSCLC-Radiomics. Other data sets in the Cancer Imaging Archive that were used in the same study published in Nature Communications: Head-Neck-Radiomics-HN1, NSCLC-Radiomics-Interobserver1, RIDER-LungCT-Seg.
Data Access
Version 1: Updated 2014/07/02
Title | Data Type | Format | Access Points | Subjects | License | |||
---|---|---|---|---|---|---|---|---|
Images | CT | DICOM | Download requires NBIA Data Retriever |
89 | 89 | 89 | 13,482 | CC BY-NC 3.0 |
Lung3 clinical | XLS | CC BY-NC 3.0 |
Additional Resources for this Dataset
The following external resources have been made available by the data submitters. These are not hosted or supported by TCIA, but may be useful to researchers utilizing this collection.
The NCI Cancer Research Data Commons (CRDC) provides access to additional data and a cloud-based data science infrastructure that connects data sets with analytics tools to allow users to share, integrate, analyze, and visualize cancer research data.
- Imaging Data Commons (IDC) (Imaging Data)
Citations & Data Usage Policy
Data Citation Required: Users must abide by the TCIA Data Usage Policy and Restrictions. Attribution must include the following citation, including the Digital Object Identifier:
Data Citation |
|
Aerts HJWL, Rios Velazquez E, Leijenaar RTH, Parmar C, Grossmann P, Carvalho S, Bussink J, Monshouwer R, Haibe-Kains B, Rietveld D, Hoebers F, Rietbergen MM, Leemans CR, Dekker A, Quackenbush J, Gillies RJ, & Lambin P. (2015). Data From NSCLC-Radiomics-Genomics. The Cancer Imaging Archive. https://doi.org/10.7937/K9/TCIA.2015.L4FRET6Z |
Detailed Description
Corresponding microarray data acquired for the imaging samples are available at National Center for Biotechnology Information (NCBI) Gene Expression Omnibus (Link to GEO: http://www.ncbi.nlm.nih.gov/geo/query/acc.cgi?acc=GSE58661). The patient names used to identify the cases on GEO are identical to those used in the DICOM files on TCIA and in the clinical data spreadsheet.
Corresponding clinical data can be found here: Lung3.metadata.xls. DICOM patients names are identical in TCIA and clinical data file.
Other Publications Using this Data
TCIA maintains a list of publications which leverage our data. If you have a manuscript you’d like to add, please contact TCIA’s Helpdesk.
- Chen, L., Qi, H., Lu, D., Zhai, J., Cai, K., Wang, L., . . . Zhang, Z. (2022). Machine vision-assisted identification of the lung adenocarcinoma category and high-risk tumor area based on CT images. Patterns (N Y), 3(4), 100464. doi: https://doi.org/10.1016/j.patter.2022.100464
- Choi, J., Cho, H. H., Kwon, J., Lee, H. Y., & Park, H. (2021). A Cascaded Neural Network for Staging in Non-Small Cell Lung Cancer Using Pre-Treatment CT. Diagnostics (Basel), 11(6). doi: https://doi.org/10.3390/diagnostics11061047
- Chui, K. T., Arya, V., Band, S. S., Alhalabi, M., Liu, R. W., & Chi, H. R. (2023). Facilitating innovation and knowledge transfer between homogeneous and heterogeneous datasets: Generic incremental transfer learning approach and multidisciplinary studies. Journal of Innovation & Knowledge, 8(2). doi: https://doi.org/10.1016/j.jik.2023.100313
- Cury, S. S., de Moraes, D., Freire, P. P., de Oliveira, G., Marques, D. V. P., Fernandez, G. J., . . . Carvalho, R. F. (2019). Tumor Transcriptome Reveals High Expression of IL-8 in Non-Small Cell Lung Cancer Patients with Low Pectoralis Muscle Area and Reduced Survival. Cancers (Basel), 11(9). doi:10.3390/cancers11091251
- Erkoc, M., & Icer, S. (2022). Analysis of Computed Tomography Images of Lung Cancer Patients with The Marker Controlled Based Method. Paper presented at the 2022 Medical Technologies Congress (TIPTEKNO), Antalya, Türkiye.
- Horng, H., Singh, A., Yousefi, B., Cohen, E. A., Haghighi, B., Katz, S., . . . Shinohara, R. T. (2022). Improved generalized ComBat methods for harmonization of radiomic features. Sci Rep, 12(1), 19009. doi:https://doi.org/10.1038/s41598-022-23328-0
- Khodabakhshi, Z., Mostafaei, S., Arabi, H., Oveisi, M., Shiri, I., & Zaidi, H. (2021). Non-small cell lung carcinoma histopathological subtype phenotyping using high-dimensional multinomial multiclass CT radiomics signature. Comput Biol Med, 136, 104752. doi: https://doi.org/10.1016/j.compbiomed.2021.104752
- Lin, P., Lin, Y. Q., Gao, R. Z., Wan, W. J., He, Y., & Yang, H. (2023). Integrative radiomics and transcriptomics analyses reveal subtype characterization of non-small cell lung cancer. Eur Radiol. doi:https://doi.org/10.1007/s00330-023-09503-5
- Pastor-Serrano, O., & Perko, Z. (2022). Millisecond speed deep learning based proton dose calculation with Monte Carlo accuracy. Phys Med Biol, 67(10). doi: https://doi.org/10.1088/1361-6560/ac692e
- Patel, D., Cowan, C., & Prasanna, P. (2021). Predicting Mutation Status and Recurrence Free Survival in Non-Small Cell Lung Cancer: A Hierarchical ct Radiomics – Deep Learning Approach. Paper presented at the 2021 IEEE 18th International Symposium on Biomedical Imaging (ISBI), Nice, France.
- Primakov, S. P., Ibrahim, A., van Timmeren, J. E., Wu, G., Keek, S. A., Beuque, M., . . . Lambin, P. (2022). Automated detection and segmentation of non-small cell lung cancer computed tomography images. Nature Communications, 13(1), 3423. doi: https://doi.org/10.1038/s41467-022-30841-3
- Trebeschi, S., Bodalal, Z., van Dijk, N., Boellaard, T. N., Apfaltrer, P., Tareco Bucho, T. M., . . . Beets-Tan, R. G. H. (2021). Development of a Prognostic AI-Monitor for Metastatic Urothelial Cancer Patients Receiving Immunotherapy. Front Oncol, 11, 637804. doi: https://doi.org/10.3389/fonc.2021.637804
- Yu, L., Tao, G., Zhu, L., Wang, G., Li, Z., Ye, J., & Chen, Q. (2019). Prediction of pathologic stage in non-small cell lung cancer using machine learning algorithm based on CT image feature analysis. BMC Cancer, 19(1), 464. doi: https://doi.org/10.1186/s12885-019-5646-9
Altmetrics
Publication Citation |
|
Aerts HJWL, Rios Velazquez E, Leijenaar RTH, Parmar C, Grossmann P, Carvalho S, Bussink J, Monshouwer R, Haibe-Kains B, Rietveld D, Hoebers F, Rietbergen MM, Leemans CR, Dekker A, Quackenbush J, Gillies RJ, & Lambin P. (2014) Decoding tumour phenotype by noninvasive imaging using a quantitative radiomics approach. Nature Communications 5, 4006 . https://doi.org/10.1038/ncomms5006 |
TCIA Citation |
|
Clark, K., Vendt, B., Smith, K., Freymann, J., Kirby, J., Koppel, P., Moore, S., Phillips, S., Maffitt, D., Pringle, M., Tarbox, L., & Prior, F. (2013). The Cancer Imaging Archive (TCIA): Maintaining and Operating a Public Information Repository. In Journal of Digital Imaging (Vol. 26, Issue 6, pp. 1045–1057). Springer Science and Business Media LLC. https://doi.org/10.1007/s10278-013-9622-7 |