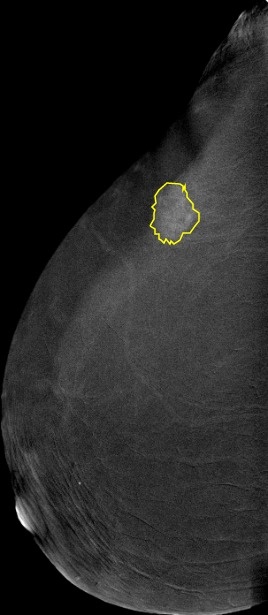
CDD-CESM | Categorized Digital Database for Low energy and Subtracted Contrast Enhanced Spectral Mammography images
DOI: 10.7937/29kw-ae92 | Data Citation Required | Image Collection
Location | Species | Subjects | Data Types | Cancer Types | Size | Status | Updated | |
---|---|---|---|---|---|---|---|---|
Breast | Human | 326 | MG | Breast Cancer | Clinical, Image Analyses, Software/Source Code | Public, Complete | 2021/12/31 |
Summary
Deep learning (DL) has a promising potential in reducing the workload of radiologists and helping them provide a more accurate diagnosis. However, fully annotated and large-sized datasets are required. This dataset is a collection of 2,006 high-resolution Contrast-enhanced spectral mammography (CESM) images with annotations and medical reports.
Acquisition protocol:
CESM is done using the standard digital mammography equipment, with additional software that performs dual-energy image acquisition. Two minutes after intravenously injecting the patient with non-ionic low-osmolar iodinated contrast material (dose: 1.5 mL/kg), craniocaudal (CC) and mediolateral oblique (MLO) views are obtained. Each view comprises two exposures, one with low energy (peak kilo-voltage values ranging from 26 to 31kVp) and one with high energy (45 to 49 kVp). Low and high-energy images are then recombined and subtracted through appropriate image processing to suppress the background breast parenchyma. A complete examination is carried out in about 5-6 minutes.
Image preprocessing:
The images were converted from DICOM to JPEG using RadiAnt with best 100% image quality (lossless). They have an average of 2355 x 1315 pixels.
Supporting data:
Full medical reports are also provided for each case (DOCX) along with manual segmentation annotation for the abnormal findings in each image (CSV file). Each image with its corresponding manual annotation (breast composition, mass shape, mass margin, mass density, architectural distortion, asymmetries, calcification type, calcification distribution, mass enhancement pattern, non-mass enhancement pattern, non-mass enhancement distribution, and overall BIRADS assessment) is compiled into 1 Excel file.
https://www.robots.ox.ac.uk/~vgg/software/via/via.html was used for the segmentation annotation. It can be used to show the annotations on the images by clicking on Annotation--> import annotations (from csv), and then proceeding to upload any image to view the annotations drawn over it. Moreover, a helper repository is created to help with pre-processing, model training, model evaluation, and segmentation annotation loading: https://github.com/omar-mohamed/CDD-CESM-Dataset
Regarding the tabs on the annotations Excel file, these are commonly used radiological descriptors as defined by the American College of Radiology 2013 lexicon.
Data Access
Version 1: Updated 2021/12/31
Title | Data Type | Format | Access Points | Subjects | License | |||
---|---|---|---|---|---|---|---|---|
Low Energy Images (0.64GB) and Subtracted Images (0.82 GB) | MG | JPG and ZIP | Download requires IBM-Aspera-Connect plugin |
326 | 2,006 | CC BY 4.0 | ||
Clinical data | DOCX and ZIP | 326 | 0 | CC BY 4.0 | ||||
Radiology hand drawn segmentations v2 | CSV | CC BY 4.0 | ||||||
Radiology manual annotations | XLSX | CC BY 4.0 |
Additional Resources for this Dataset
The following external resources have been made available by the data submitters. These are not hosted or supported by TCIA, but may be useful to researchers utilizing this collection.
- Source Code : https://github.com/omar-mohamed/CDD-CESM-Dataset
- Segmentation annotation code: https://www.robots.ox.ac.uk/~vgg/software/via/via.html
Citations & Data Usage Policy
Data Citation Required: Users must abide by the TCIA Data Usage Policy and Restrictions. Attribution must include the following citation, including the Digital Object Identifier:
Data Citation |
|
Khaled R., Helal M., Alfarghaly O., Mokhtar O., Elkorany A., El Kassas H., Fahmy A. Categorized Digital Database for Low energy and Subtracted Contrast Enhanced Spectral Mammography images [Dataset]. (2021) The Cancer Imaging Archive. DOI: 10.7937/29kw-ae92 |
Acknowledgements
We would like to acknowledge the individuals and institutions that have provided data for this collection:
- National Cancer Institute, Cairo University, Cairo, Egypt : Special thanks to Dr. Rana Khaled, M.Sc, Prof. Maha Helal, MD, Prof. Omnia Mokhtar, MD and Dr. Hebatalla El Kassas, MD from the Department of Radiology.
- Faculty of Computers and Artificial Intelligence, Cairo University, Cairo, Egypt – Special thanks to Omar Alfarghaly, Prof. Abeer Elkorany, and Prof. Aly Fahmy from the Department of Computer Science.
Other Publications Using this Data
TCIA maintains a list of publications which leverage TCIA data. If you have a manuscript you’d like to add please contact TCIA’s Helpdesk.
Publication Citation |
|
Khaled, R., Helal, M., Alfarghaly, O., Mokhtar, O., Elkorany, A., El Kassas, H., & Fahmy, A. Categorized contrast enhanced mammography dataset for diagnostic and artificial intelligence research. (2022) Scientific Data, Volume 9, Issue 1. DOI: 10.1038/s41597-022-01238-0 |
TCIA Citation |
|
Clark, K., Vendt, B., Smith, K., Freymann, J., Kirby, J., Koppel, P., Moore, S., Phillips, S., Maffitt, D., Pringle, M., Tarbox, L., & Prior, F. (2013). The Cancer Imaging Archive (TCIA): Maintaining and Operating a Public Information Repository. In Journal of Digital Imaging (Vol. 26, Issue 6, pp. 1045–1057). Springer Science and Business Media LLC. https://doi.org/10.1007/s10278-013-9622-7 |