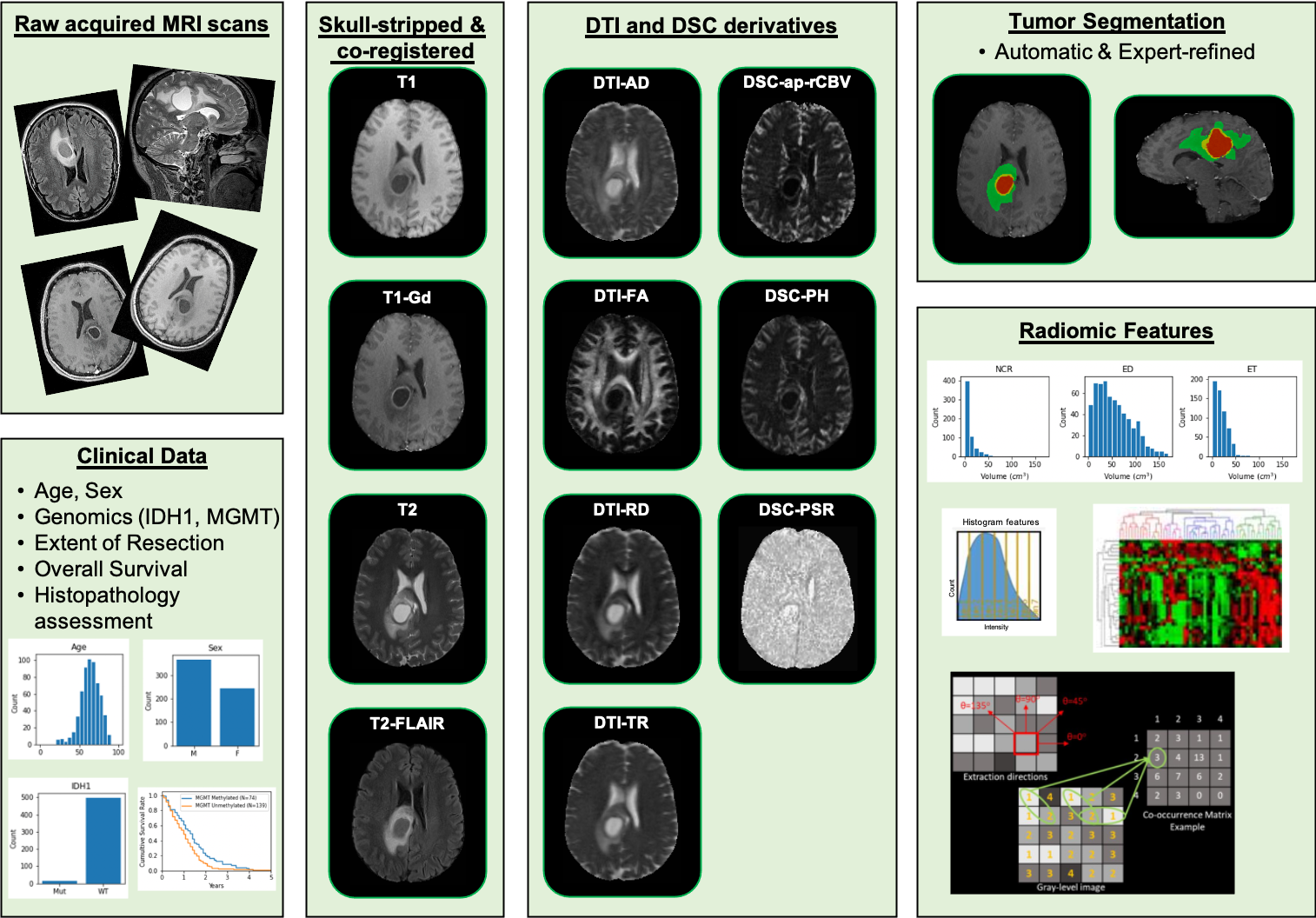
UPENN-GBM | Multi-parametric magnetic resonance imaging (mpMRI) scans for de novo Glioblastoma (GBM) patients from the University of Pennsylvania Health System
DOI: 10.7937/TCIA.709X-DN49 | Data Citation Required | Image Collection
Location | Species | Subjects | Data Types | Cancer Types | Size | Status | Updated | |
---|---|---|---|---|---|---|---|---|
Brain | Human | 630 | MR, Histopathology | Glioblastoma | Clinical, Image Analyses | Public, Complete | 2022/10/24 |
Summary
This collection comprises multi-parametric magnetic resonance imaging (mpMRI) scans for de novo Glioblastoma (GBM) patients from the University of Pennsylvania Health System, coupled with patient demographics, clinical outcome (e.g., overall survival, genomic information, tumor progression), as well as computer-aided and manually-corrected segmentation labels of multiple histologically distinct tumor sub-regions, computer-aided and manually-corrected segmentations of the whole brain, a rich panel of radiomic features along with their corresponding co-registered mpMRI volumes in NIfTI format. Scans were initially skull-stripped and co-registered, before their tumor segmentation labels were produced by an automated computational method. These segmentation labels were revised and any label misclassifications were manually corrected/approved by expert board-certified neuroradiologists. The final labels were used to extract a rich panel of imaging features, including intensity, volumetric, morphologic, histogram-based and textural parameters. The segmentation labels enable quantitative computational and clinical studies without the need to repeat manual annotations whilst allowing for comparison across studies. They can also serve as a set of manually-annotated gold standard labels for performance evaluation in computational challenges. The provided panel of radiomic features may facilitate research integrative of the molecular characterization offered, and hence allow associations with molecular markers (radiogenomic biomarker research), clinical outcomes, treatment responses and other endpoints, by researchers without sufficient computational background to extract such features. Additional data accompanying the UPENN-GBM data collection include H&E-stained digitized tissue sections from resected tumor specimens of matched de novo and recurrent cases for a few of the patients in this collection.
Data Access
Version 2: Updated 2022/10/24
changes: Histopathology NDPI slides added to collection. CSV file for mapping Radiology subject IDs to Histopathology patient and image IDs where available (note: not all Radiology data has associated pathology data and vice versa).
Title | Data Type | Format | Access Points | Subjects | License | |||
---|---|---|---|---|---|---|---|---|
Images | MR | DICOM | Download requires NBIA Data Retriever |
630 | 3,301 | 3,680 | 828,234 | CC BY 4.0 |
Images | NIFTI | Download requires IBM-Aspera-Connect plugin |
CC BY 4.0 | |||||
Histopathology Images | Histopathology | NDPI | Download requires IBM-Aspera-Connect plugin |
34 | 71 | CC BY 4.0 | ||
Clinical Data | CSV | 671 | CC BY 4.0 | |||||
Histopathology to Radiology Filename Mapping | CSV | CC BY 4.0 | ||||||
Image acquisition parameters | CSV | CC BY 4.0 | ||||||
Data availability per subject | CSV | CC BY 4.0 | ||||||
CaPTk radiomic features list | CSV | CC BY 4.0 | ||||||
CaPTk radiomic feature parameter file | CSV | CC BY 4.0 | ||||||
Radiomic Data | ZIP | CC BY 4.0 |
Additional Resources for this Dataset
The NCI Cancer Research Data Commons (CRDC) provides access to additional data and a cloud-based data science infrastructure that connects data sets with analytics tools to allow users to share, integrate, analyze, and visualize cancer research data.
- Imaging Data Commons (IDC) (Imaging Data)
Citations & Data Usage Policy
Data Citation Required: Users must abide by the TCIA Data Usage Policy and Restrictions. Attribution must include the following citation, including the Digital Object Identifier:
Data Citation |
|
Bakas, S., Sako, C., Akbari, H., Bilello, M., Sotiras, A., Shukla, G., Rudie, J. D., Flores Santamaria, N., Fathi Kazerooni, A., Pati, S., Rathore, S., Mamourian, E., Ha, S. M., Parker, W., Doshi, J., Baid, U., Bergman, M., Binder, Z. A., Verma, R., … Davatzikos, C. (2021). Multi-parametric magnetic resonance imaging (mpMRI) scans for de novo Glioblastoma (GBM) patients from the University of Pennsylvania Health System (UPENN-GBM) (Version 2) [Data set]. The Cancer Imaging Archive. https://doi.org/10.7937/TCIA.709X-DN49 |
Detailed Description
Note from the submitting group: The NIfTI images are all registered to a common atlas (SRI) using a uniform preprocessing and the segmentation are aligned with them. Therefore the NIfTI images will not align with the DICOM images, by design. If you load the NIfTI images (like T1/T2) and their related segmentation, these will line up.
Acknowledgements
Reported research was partly supported by the National Cancer Institute (NCI), the National Institute of Neurological Disorders and Stroke (NINDS), and the National Center for Advancing Translational Sciences (NCATS) of the National Institutes of Health (NIH) under award numbers NINDS:R01NS042645, NCI:U24CA189523, NCI:U01CA242871, NCATS:UL1TR001878, and by the Institute for Translational Medicine and Therapeutics (ITMAT) of the University of Pennsylvania. The content of this publication is solely the responsibility of the authors and does not represent the official views of the NIH, or the ITMAT of the UPenn.
Related Publications
Publication Citation |
|
Bakas, S., Sako, C., Akbari, H., Bilello, M., Sotiras, A., Shukla, G., Rudie, J. D., Flores Santamaria, N., Fathi Kazerooni, A., Pati, S., Rathore, S., Mamourian, E., Ha, S. M., Parker, W., Doshi, J., Baid, U., Bergman, M., Binder, Z. A., Verma, R., Lustig, R., Desai, A. S., Bagley, S. J., Mourelatos, Z., Morrissette, J., Watt, C. D., Brem, S., Wolf, R. L., Melhem, E. R., Nasrallah, M. P., Mohan, S., O’Rourke, D. M., Davatzikos, C. (2022). The University of Pennsylvania glioblastoma (UPenn-GBM) cohort: advanced MRI, clinical, genomics, & radiomics. In Scientific Data (Vol. 9, Issue 1). https://doi.org/10.1038/s41597-022-01560-7 |
Research Community Publications
TCIA maintains a list of publications which leverage TCIA data. If you have a manuscript you’d like to add please contact TCIA’s Helpdesk.
Previous Versions
Version 1: Updated 2022/06/21
Title | Data Type | Format | Access Points | License | ||||
---|---|---|---|---|---|---|---|---|
Images | DICOM | Download requires NBIA Data Retriever |
CC BY 4.0 | |||||
Images | NIFTI | Download requires IBM-Aspera-Connect plugin |
CC BY 4.0 | |||||
Clinical Data | CSV | CC BY 4.0 | ||||||
Image acquisition parameters | CSV | CC BY 4.0 | ||||||
Data availability per subject | CSV | CC BY 4.0 | ||||||
CaPTk radiomic features list | CSV | CC BY 4.0 | ||||||
CaPTk radiomic feature parameter file | CSV | CC BY 4.0 | ||||||
Radiomic Data | ZIP | CC BY 4.0 | ||||||
CaPTk radiomic feature parameter | CSV | CC BY 4.0 |