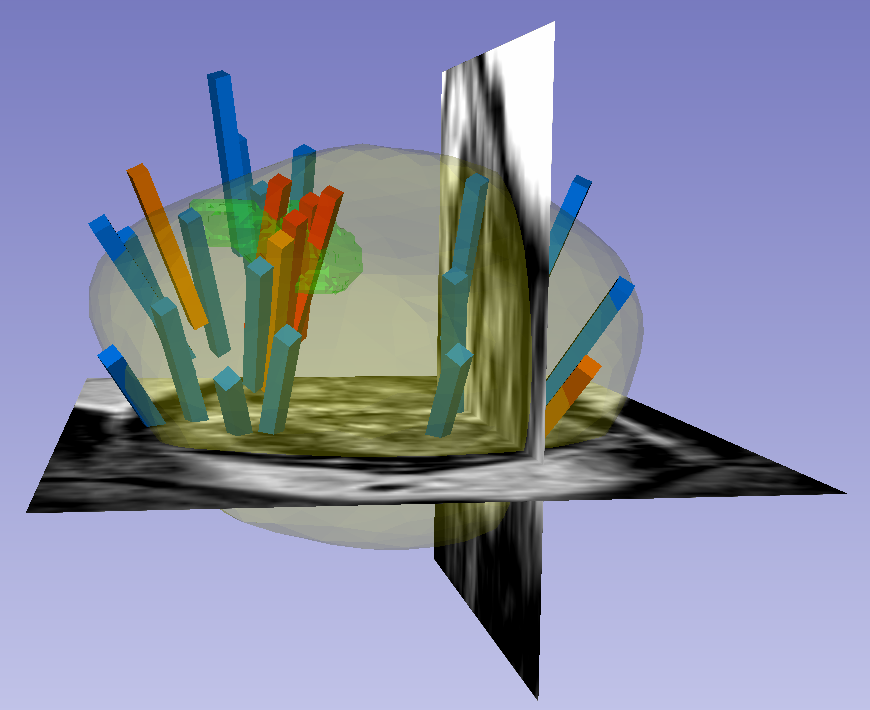
Prostate-MRI-US-Biopsy | Prostate MRI and Ultrasound With Pathology and Coordinates of Tracked Biopsy
DOI: 10.7937/TCIA.2020.A61IOC1A | Data Citation Required | Image Collection
Location | Species | Subjects | Data Types | Cancer Types | Size | Status | Updated | |
---|---|---|---|---|---|---|---|---|
Prostate | Human | 1,151 | US, MR | Prostate Cancer | Image Analyses | Public, Complete | 2023/10/20 |
Summary
This dataset was derived from tracked biopsy sessions using the Artemis biopsy system, many of which included image fusion with MRI targets. Patients received a 3D transrectal ultrasound scan, after which nonrigid registration (e.g. “fusion”) was performed between real-time ultrasound and preoperative MRI, enabling biopsy cores to be sampled from MR regions of interest. Most cases also included sampling of systematic biopsy cores using a 12-core digital template. The Artemis system tracked targeted and systematic core locations using encoder kinematics of a mechanical arm, and recorded locations relative to the Ultrasound scan. MRI biopsy coordinates were also recorded for most cases. STL files and biopsy overlays are available and can be visualized in 3D Slicer with the SlicerHeart extension. Spreadsheets summarizing biopsy and MR target data are also available. See the Detailed Description tab below for more information. MRI targets were defined using multiparametric MRI, e.g. t2-weighted, diffusion-weighted, and perfusion-weighted sequences, and scored on a Likert-like scale with close correspondence to PIRADS version 2. t2-weighted MRI was used to trace ROI contours, and is the only sequence provided in this dataset. MR imaging was performed on a 3 Tesla Trio, Verio or Skyra scanner (Siemens, Erlangen, Germany). A transabdominal phased array was used in all cases, and an endorectal coil was used in a subset of cases. The majority of pulse sequences are 3D T2:SPC, with TR/TE 2200/203, Matrix/FOV 256 × 205/14 × 14 cm, and 1.5mm slice spacing. Some cases were instead 3D T2:TSE with TR/TE 3800–5040/101, and a small minority were imported from other institutions (various T2 protocols.) Ultrasound scans were performed with Hitachi Hi-Vision 5500 7.5 MHz or the Noblus C41V 2-10 MHz end-fire probe. 3D scans were acquired by rotation of the end-fire probe 200 degrees about its axis, and interpolating to resample the volume with isotropic resolution. Patients with suspicion of prostate cancer due to elevated PSA and/or suspicious imaging findings were consecutively accrued. Any consented patient who underwent or had planned to receive a routine, standard-of-care prostate biopsy at the UCLA Clark Urology Center was included. Note: Some Private Tags in this collection are critical to properly displaying the STL surface and the Prostate anatomy. Private Tag (1129,"Eigen, Inc",1016) DS VoxelSize is especially important for multi-frame US cases.
Data Access
Version 2: Updated 2023/10/20
Added Diffusion-weighted imaging for 837 participants.
Title | Data Type | Format | Access Points | Subjects | License | |||
---|---|---|---|---|---|---|---|---|
Images | US, MR | DICOM | Download requires NBIA Data Retriever |
1,151 | 2,799 | 4,819 | 102,397 | CC BY 4.0 |
Target Data | XLSX | CC BY 4.0 | ||||||
Biopsy Data | XLSX | CC BY 4.0 | ||||||
STL Files | ZIP | Download requires IBM-Aspera-Connect plugin |
CC BY 4.0 | |||||
Biopsy Overlays | ZIP | Download requires IBM-Aspera-Connect plugin |
CC BY 4.0 |
Additional Resources for this Dataset
The NCI Cancer Research Data Commons (CRDC) provides access to additional data and a cloud-based data science infrastructure that connects data sets with analytics tools to allow users to share, integrate, analyze, and visualize cancer research data.
- Imaging Data Commons (IDC) (Imaging Data)
- IDC Zenodo community dataset DICOM converted annotations for the Prostate-MRI-US-Biopsy collection
Citations & Data Usage Policy
Data Citation Required: Users must abide by the TCIA Data Usage Policy and Restrictions. Attribution must include the following citation, including the Digital Object Identifier:
Data Citation |
|
Natarajan, S., Priester, A., Margolis, D., Huang, J., & Marks, L. (2020). Prostate MRI and Ultrasound With Pathology and Coordinates of Tracked Biopsy (Prostate-MRI-US-Biopsy) (version 2) [Data set]. The Cancer Imaging Archive. DOI: 10.7937/TCIA.2020.A61IOC1A |
Detailed Description
Instructions for Proper Use of the STL Files
The 3D surfaces of this dataset are in stereolithography format (binary representation). They are segmentations representing anatomic regions within the corresponding ultrasound (US) or MRI images. Each file is named “Prostate-MRI-US-Biopsy-XXXX-SURFACETYPE-seriesUID-YYYY.STL” where “XXXX” is the anonymized patient number and “YYYY” is the series instance UID of corresponding DICOM images. “SURFACETYPE” can be one of two identifiers, summarized below.
- If “SURFACETYPE” is “ProstateSurface,” then the STL file is a segmentation of the prostate gland. These were contoured semi-automatically with Profuse (for MRI) or the Artemis fusion biopsy system (for US), both products of Eigen (Grass Valley, CA). Using these tools, the user manually traced the prostate on one or more image planes, and the remainder of gland was automatically segmented in 3D. The user was then able to adjust vertices of the 3D segmentation as necessary. These segmentations are generally of good quality, though their accuracy is user-dependent.
- If “SURFACETYPE” is “Target,” then the STL file is a segmentation of a Region of Interest (ROI) suspicious for harboring prostate cancer. Zero, one, or more than one ROI may be present, and thus are designated “Target1,” “Target2,” etc. The ROIs corresponding to MRI were traced manually by a radiologist based on their interpretation of the patient’s multiparametric MRI study. The ROIs corresponding to US images were acquired by registering the MRI prostate surface nonrigidly and semi-automatically to the US prostate surface. Therefore, the US ROIs are subject to misregistration, with average accuracy of 3-4 mm.
- Note that proximity to an ROI increases the likelihood of, but does not guarantee, prostate cancer. Also note that radiologists assigned each ROI a Likert-like suspicion score of 1-5, with 1 corresponding to very low suspicion of prostate cancer and 5 corresponding to very high suspicion of prostate cancer. These scores, which are roughly equivalent to the “PIRADS Version 2” scoring system, can be found in a spreadsheet included with the dataset.
The STL files can be visualized in 3D Slicer as follows:
- Import the surface(s) either by drag-and-dropping or with File->Add Data
- Within the “Models” module, adjust Display->Opacity of the prostate surface in order to visualize any ROI(s) within
- To visualize surfaces in the context of corresponding MRI or US images, load the corresponding image series and then toggle the Slice Display->Visible radio button within the “models” module
- To visualize surfaces in the context of biopsy cores, import the corresponding 3D slicer file named “BiopsyVectors.mrml” by drag-and-dropping or with File->Add Data.
The STL files, which are written in a standard format, can also be imported and visualized with most computer-aided design (CAD) and 3D printing software. Note that, if quantitative analysis is desired, the following transform was applied to US surfaces for 3D slicer compatibility and may need to be reversed for visualization alongside MRI or US images using other software.
Transform (which has been applied to all ultrasound data):
[0 1 0
0 0 1
1 0 0]
Inverse Transform (use to revert ultrasound data to original coordinates):
[0 0 1
1 0 0
0 1 0]
Instructions for Proper Use of the “Biopsy Overlay” Files
Biopsy vectors, prostate surfaces, and regions of interest can be imported to 3D Slicer in a single step using the collection’s “Biopsy Overlay” files. Overlay files associated with ultrasound images are organized into folders with the naming convention “Prostate-MRI-US-Biopsy-XXXX-BXus-seriesUID-####” where “XXXX” is the anonymized patient number and “####” is the series instance UID of corresponding DICOM images. Files associated with MR images have an analogous naming convention “Prostate-MRI-US-Biopsy-XXXX-BXmr-seriesUID-####”, but are appended with “(US-date-YYYYMMDD)” where ‘YYYYMMDD’ is the de-identified acquisition date of the ultrasound series registered to MRI during biopsy. This is specified because in some cases multiple ultrasound-guided biopsies were registered to the same MRI series.
Within each folder of “Biopsy Overlays” is a file named “BiopsyVectors.mrml”. This can be imported directly into 3D Slicer by either by drag-and-dropping or with File->Add Data. The prostate surface, any ROIs present, and all biopsy vectors will then be displayed within 3D Slicer. The biopsy cores, represented as “line” fiducials, are color-coded as BLUE = benign, ORANGE = Gleason Grade 3+3, and RED = Gleason Grade ≥ 3+4. The prostate is shown as WHITE, and ROIs are GREEN. To visualize these overlays in the context of corresponding MRI or US images, load the corresponding image series to 3D Slicer using File->DICOM.
Instructions for Proper Use of the Biopsy and Target Spreadsheets
Spreadsheets accompany the dataset that summarize biopsy and MRI target information.
In the spreadsheet “TCIA Biopsy Data,” each row represents a single core acquired during ultrasound-guided biopsy. This information includes:
- Coordinates of the biopsy core relative to ultrasound images
- Coordinates of the biopsy core relative to MR images. These are present for approximately 70% of the dataset; values of “-1000” are placeholders representing missing data.
- Primary and secondary Gleason score of the biopsy core. Blank entries represent cores containing only benign tissue.
- Length of cancer (mm) within the biopsy core. Blank entries represent cores containing only benign tissue.
- Total tissue length (mm) of the biopsy core; multiple lengths are listed for cores that were fragmented. Tissue lengths are present for approximately 60% of the dataset; blank entries represent cores for which total tissue length was not recorded.
- Core labels (textual description).
- Prostate serum antigen of patient at the time of biopsy.
- Prostate volume of the patient at the time of biopsy, as measured via MRI prostate segmentation.
- De-identified patient number, series instance UID of ultrasound, and series instance UID of MR images associated with the biopsy core. Note that MRI UIDs are present for approximately 70% of the dataset; blank entries represent cases for which data was irretrievable or MRI registration was not performed.
In the spreadsheet “Target Data,” each row represents a single region of interest (ROI), corresponding to one of the exported Target STL files. This information includes:
- Likert-Like “UCLA” score of the ROI. This score predates but is similar to “PIRADSv2.” Score values range from 1-5, where 1 is very low suspicion of prostate cancer and 5 is very high suspicion of prostate cancer. Note that scores of 0 or 1 may represent segmentation of non-malignant morphology, or else cases for which a score could not be properly assigned. Only scores of 2 or higher should be considered to correlate with risk of prostate cancer.
- Volume (in CCs) of the ROI.
- Target number of the ROI, enabling correlation between STL surfaces and the suspicion scores for cases with multiple targets.
- De-identified patient number and series instance UID of MR images associated with the ROI.
Diffusion-MRI-Supplement-To-Prostate-MRI-US-Biopsy
The following diffusion-weighted MRI series are provided for 837 participants present in the “Prostate-MRI-US-Biopsy” dataset:
- Apparent Diffusion Coefficient (ADC)
- High B-value DWI, most commonly with a calculated B-value of 1400
Most commonly these series were generated from DWI images with B-values of [0, 100, 400, 800], consist of 20 image slices, have a resolution of approximately 1.6 x 1.6 x 3.6 mm, and include a window size of the pelvis measuring approximately 256 x 210 mm.
Some of the ADC imaging here were created at the scanner, though some were processed later through MATLAB offline to produce a uniform set of ADC pixel data to supplement this collection. Note: Some (0019,xxxx) and (0043,xxxx) tag content may have been mangled in this external processing step, but the images should be consistent.
Thus, the T2-weighted MRI series from version 1 of this Collection are supplemented by ADC and high B-value images acquired during the same study, enabling bi-parametric analysis and PI-RADS assessment of regions of interest (ROIs).
Scientific questions that could be answered using this data include:
- What is the optimum ADC value associated with cancer positivity of prostate biopsy cores
- What is the optimum weighting of diffusion MRI versus T2 in ROI grading (using biopsy as ground-truth for prostate cancer)?
- Can diffusion MRI be used to help an AI model automatically identify and/or segment ROIs suspicious for prostate cancer?
No other public dataset provides both bi-parametric prostate MRI and tracked biopsy pathology. In addition, this data could be of substantial value to participants in the “PI-CAI” challenge, the goal of which is to develop AI models for patient-level diagnosis and lesion-level detection of prostate cancer using bi-parametric MRI.
Acknowledgements
We would like to acknowledge the individuals and institutions that have provided data for this collection:
- This work was supported in part by the National Cancer Institute Award R01CA158627, Prostate Cancer SPORE at University of California-Los Angeles P50CA092131, the Beckman Coulter Foundation, Jean Perkins Foundation, and Steven C. Gordon Family Foundation
- Harmonization of the components of this dataset, including into standard DICOM representation, was supported in part by the NCI Imaging Data Commons consortium. NCI Imaging Data Commons consortium is supported by the contract number 19X037Q from Leidos Biomedical Research under Task Order HHSN26100071 from NCI
Related Publications
Publications by the Dataset Authors
The Collection authors suggest the below will give context to this dataset:
- Elkhoury, F. F., Felker, E. R., Kwan, L., Sisk, A. E., Delfin, M., Natarajan, S., & Marks, L. S. (2019). Comparison of Targeted vs Systematic Prostate Biopsy in Men Who Are Biopsy Naive. In JAMA Surgery (Vol. 154, Issue 9, p. 811). American Medical Association (AMA). https://doi.org/10.1001/jamasurg.2019.1734
- Jayadevan, R., Felker, E. R., Kwan, L., Barsa, D. E., Zhang, H., Sisk, A. E., Delfin, M., & Marks, L. S. (2019). Magnetic Resonance Imaging–Guided Confirmatory Biopsy for Initiating Active Surveillance of Prostate Cancer. In JAMA Network Open (Vol. 2, Issue 9, p. e1911019). American Medical Association (AMA). https://doi.org/10.1001/jamanetworkopen.2019.11019
- Simopoulos, D. N., Sisk, A. E., Jr., Priester, A., Felker, E. R., Kwan, L., Delfin, M. K., Reiter, R. E., & Marks, L. S. (2019). Cancer core length from targeted biopsy: an index of prostate cancer volume and pathological stage. In BJU International (Vol. 124, Issue 2, pp. 275–281). Wiley. https://doi.org/10.1111/bju.14691
- Kinnaird, A., Sharma, V., Chuang, R., Priester, A., Tran, E., Barsa, D. E., Delfin, M., Kwan, L., Sisk, A., Felker, E., & Marks, L. S. (2020). Risk of Prostate Cancer after a Negative Magnetic Resonance Imaging Guided Biopsy. In Journal of Urology (Vol. 204, Issue 6, pp. 1180–1186). Ovid Technologies (Wolters Kluwer Health). https://doi.org/10.1097/ju.0000000000001232
- Zhou, S. R., Chang, E., Patankar, A., Huang, J., Marks, L. S., & Natarajan, S. (2020). Prostate Cancer Detection Rate of Freehand versus 3-Dimensional Template Mapping Biopsy Using a Magnetic Resonance Imaging-Ultrasound Fusion Device in Biopsy Naïve Men. In Journal of Urology (Vol. 203, Issue 4, pp. 699–705). Ovid Technologies (Wolters Kluwer Health). https://doi.org/10.1097/ju.0000000000000587
- Raman, A. G., Sarma, K. V., Raman, S. S., Priester, A. M., Mirak, S. A., Riskin-Jones, H. H., Dhinagar, N., Speier, W., Felker, E., Sisk, A. E., Lu, D., Kinnaird, A., Reiter, R. E., Marks, L. S., & Arnold, C. W. (2021). Optimizing Spatial Biopsy Sampling for the Detection of Prostate Cancer. In Journal of Urology (Vol. 206, Issue 3, pp. 595–603). Ovid Technologies (Wolters Kluwer Health). https://doi.org/10.1097/ju.0000000000001832
- Brisbane, W. G., Priester, A. M., Ballon, J., Kwan, L., Delfin, M. K., Felker, E. R., Sisk, A. E., Hu, J. C., & Marks, L. S. (2022). Targeted Prostate Biopsy: Umbra, Penumbra, and Value of Perilesional Sampling. In European Urology (Vol. 82, Issue 3, pp. 303–310). Elsevier BV. https://doi.org/10.1016/j.eururo.2022.01.008
- Hassan, Md. R., Islam, Md. F., Uddin, Md. Z., Ghoshal, G., Hassan, M. M., Huda, S., & Fortino, G. (2022). Prostate cancer classification from ultrasound and MRI images using deep learning based Explainable Artificial Intelligence. In Future Generation Computer Systems (Vol. 127, pp. 462–472). Elsevier BV. https://doi.org/10.1016/j.future.2021.09.030
- Padasdao, B., Batsaikhan, Z., Lafreniere, S., Rabiei, M., & Konh, B. (2022). Modeling and Operator Control of a Robotic Tool for Bidirectional Manipulation in Targeted Prostate Biopsy. In 2022 International Symposium on Medical Robotics (ISMR). 2022 International Symposium on Medical Robotics (ISMR). IEEE. https://doi.org/10.1109/ismr48347.2022.9807514
- Priester, A., Fan, R. E., Shubert, J., Rusu, M., Vesal, S., Shao, W., Khandwala, Y. S., Marks, L. S., Natarajan, S., & Sonn, G. A. (2023). Prediction and Mapping of Intraprostatic Tumor Extent with Artificial Intelligence. In European Urology Open Science (Vol. 54, pp. 20–27). Elsevier BV. https://doi.org/10.1016/j.euros.2023.05.018
- Vesal, S., Gayo, I., Bhattacharya, I., Natarajan, S., Marks, L. S., Barratt, D. C., Fan, R. E., Hu, Y., Sonn, G. A., & Rusu, M. (2022). Domain generalization for prostate segmentation in transrectal ultrasound images: A multi-center study. In Medical Image Analysis (Vol. 82, p. 102620). Elsevier BV. https://doi.org/10.1016/j.media.2022.102620
Publication Citation |
|
Sonn GA, Natarajan S, Margolis DJ, MacAiran M, Lieu P, Huang J, Dorey FJ, Marks LS. Targeted biopsy in the detection of prostate cancer using an office based magnetic resonance ultrasound fusion device. Journal of Urology 189, no. 1 (2013): 86-91. DOI: 10.1016/j.juro.2012.08.095 |
Research Community Publications
TCIA maintains a list of publications which leverage TCIA data. If you have a manuscript you’d like to add please contact the TCIA Helpdesk.
- Hassan, M. R., Islam, M. F., Uddin, M. Z., Ghoshal, G., Hassan, M. M., Huda, S., & Fortino, G. (2022). Prostate cancer classification from ultrasound and MRI images using deep learning based Explainable Artificial Intelligence. Future Generation Computer Systems, 127, 462-472. doi: https://doi.org/10.1016/j.future.2021.09.030
- Kosareva, A. A., Paulenka, D. A., Snezhko, E. V., Bratchenko, I. A., & Kovalev, V. A. (2022). Examining the Validity of Input Lung CT Images Submitted to the AI-Based Computerized Diagnosis. Journal of Biomedical Photonics & Engineering, 8(3). doi:https://doi.org/10.18287/JBPE22.08.030307
- Li, Y., Wang, J., Hu, M., Patel, P., Mao, H., Liu, T., . . . Chen, W. (2023). Prostate gleason score prediction via MRI using capsule network. Paper presented at the Medical Imaging 2023: Computer-Aided Diagnosis.
- Palladino, L., Maris, B., Antonelli, A., & Fiorini, P. (2022). PROST-Net: A Deep Learning Approach to Support Real-Time Fusion in Prostate Biopsy. IEEE Transactions on Medical Robotics and Bionics, 4(2), 323-326. doi: https://doi.org/10.1109/TMRB.2022.3145667
Previous Versions
Version 1: Updated 2020/09/17
Title | Data Type | Format | Access Points | License | ||||
---|---|---|---|---|---|---|---|---|
Images | DICOM | Download requires NBIA Data Retriever |
||||||
Target Data | XLSX | |||||||
Biopsy Data | XLSX | |||||||
STL Files | ZIP | Download requires IBM-Aspera-Connect plugin |
||||||
Biopsy Overlays | ZIP | Download requires IBM-Aspera-Connect plugin |