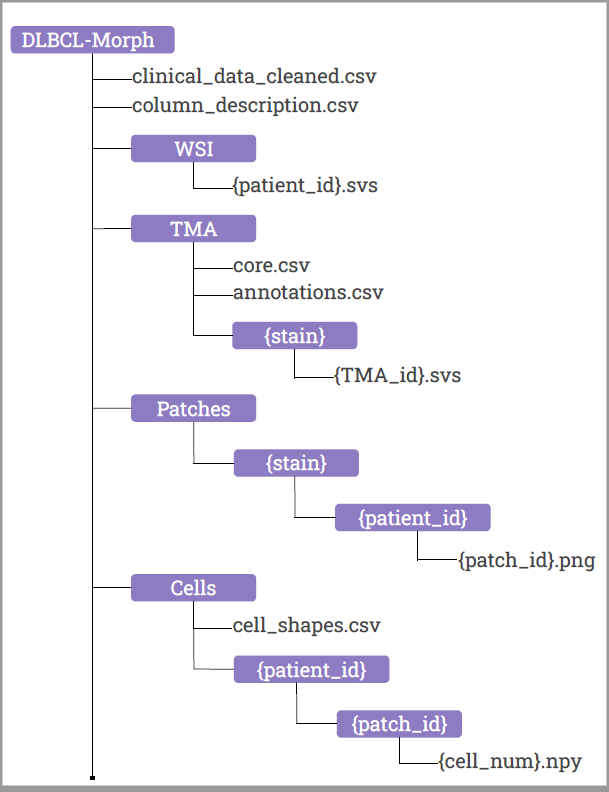
DLBCL-Morphology | H&E and immunohistochemical stain images of 209 cases of diffuse large B-cell lymphoma linked with cytogenetic features and clinical outcomes
DOI: 10.7937/NVA3-N783 | Data Citation Required | 1.4k Views | 1 Citations | Image Collection
Location | Species | Subjects | Data Types | Cancer Types | Size | Status | Updated | |
---|---|---|---|---|---|---|---|---|
Lymph Node | Human | 209 | Histopathology, Whole Slide Image, Tissue Microarray, Follow-Up, Molecular Test, Immunohistochemistry, Diagnosis, Other | Diffuse Large B-Cell Lymphoma | Clinical, Image Analyses | Public, Complete | 2022/03/25 |
Summary
Diffuse Large B-Cell Lymphoma (DLBCL) is the most common non-Hodgkin lymphoma worldwide. DLBCL is fatal without treatment, but early detection and therapy can cure up to 70% of patients. The current best prognostic classification, the National Comprehensive Cancer Network International Prognostic Index, is insufficient to guide therapeutic decision-making for individual patients. No tumor-intrinsic prognostication method is currently available. The DLBCL-Morph dataset contains 42 digital high-magnification scans of tissue microarrays (TMAs) containing tissue cores from 209 DLBCL cases at Stanford Hospital. Each DLBCL case is accompanied by survival data, follow-up status and a wide variety of clinical and cytogenetic variables. The TMAs are stained for H&E, which shows cell morphology, as well as for the expression of several prognostically relevant proteins: CD10, BCL6, MUM1, BCL2, and MYC. The TMAs are accompanied by pathologist-annotated regions of interest (ROIs) that specify areas of tissue representative of DLBCL. We used deep learning to segment out cancerous nuclei from the ROIs, and computed several geometric features for each cancerous nucleus, which are provided as part of our dataset. These geometric features quantify several morphologic properties of a nucleus, such as size and elongation, and can be used as input for automated prognostic models to predict survival. In addition, DLBCL-Morph contains 204 digital high-magnification whole-slide images (WSIs) from 149 DLBCL cases, stained for H&E. A total of 152,194 patches (240x240 pixels each) were extracted from the H&E stained ROIs and a HoVer-Net model was used to segment tumor nuclei (1,035,909 binary masks). Geometric descriptors were computed for each segmented nucleus and a Cox proportional hazards model was evaluated using A) only clinical features, B) only morphologic features, or C) both sets of features. The Cox model achieved a concordance index of A) 0.703 (p = 0.005) B) 0.645 (p = 0.07), and C) 0.723 (p < 0.001) on a randomly sampled validation set of 51 patients. Our findings suggest that a risk calculator based on both clinical and morphologic data could yield improved prognostic value for DLBCL without the need for additional diagnostic testing. Several studies have thus far failed to conclusively demonstrate that morphologic classification can predict outcomes in DLBCL. Automated medical imaging methods on whole slide images (WSI) could potentially identify novel, prognostically significant morphological or immunohistochemical biomarkers. The ability of automated methods to identify prognostically relevant features on H&E sections that have eluded pathologists has been demonstrated (Beck et al Science Translational Med). Furthermore, if successful, automated image analysis could potentially be scaled up into a cost-effective alternative to current classification methods which are typically costly and/or labor intensive. A critical requirement for the development of such deep learning models is the availability of datasets containing WSIs appropriately stained to show cell morphology and oncogene expression, with accompanying prognostic outcome data.
Data Access
Version 1: Updated 2022/03/25
Title | Data Type | Format | Access Points | Subjects | License | Metadata | |||
---|---|---|---|---|---|---|---|---|---|
Tissue Slide Images | Histopathology, Whole Slide Image, Tissue Microarray | SVS and PNG | Download requires IBM-Aspera-Connect plugin |
209 | 246 | CC BY-NC 4.0 | — | ||
Clinical data | Follow-Up, Molecular Test, Immunohistochemistry, Diagnosis | CSV | CC BY-NC 4.0 | — | |||||
Clinical data column descriptions | Other | CSV | CC BY-NC 4.0 | — |
Citations & Data Usage Policy
Data Citation Required: Users must abide by the TCIA Data Usage Policy and Restrictions. Attribution must include the following citation, including the Digital Object Identifier:
Data Citation |
|
Fernandez-Pol, S., Natkunam, Y., Vrabac, D., Rojansky, R., Advani, R., Rajpurkar, P., S, & Ng, Andrew Y. (2022). H&E and immunohistochemical stain images of 209 cases of diffuse large B-cell lymphoma linked with cytogenetic features and clinical outcomes (Version 1) [Data set]. The Cancer Imaging Archive. https://doi.org/10.7937/NVA3-N783 |
Detailed Description
Additional information about TM folder in dataset
Each row in the core.csv corresponds to a TMA core where ‘patient_id’ corresponds to the id of the patient the core belongs to. The columns ’tma_id’, ‘row’, and ‘col’ are used for locating the core in the TMA where ‘row’ and ‘col’ refers to the row and column location of the core in the TMA file with filename ’tma_id’.
In the annotations.csv file, the columns xs, ys, xe, & ye are coordinates (’s’ and ‘e’ abbreviated for ’start’ and ‘end’, respectively) where (xs, ys) and (xe, ye) is the xy-coordinates of the upper left corner and lower right corner of the annotation respectively.
Related Publications
Publications by the Dataset Authors
The authors recommended the following as the best source of additional information about this dataset:
No other publications were recommended by dataset authors.
Research Community Publications
TCIA maintains a list of publications that leveraged this dataset. If you have a manuscript you’d like to add please contact TCIA’s Helpdesk.