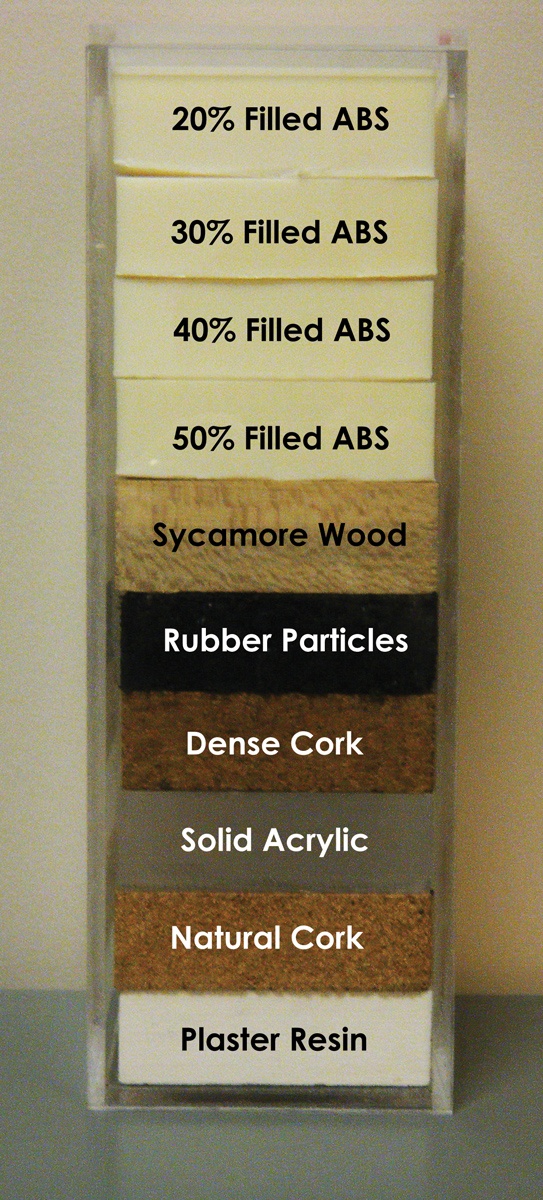
CC-Radiomics-Phantom | Credence Cartridge Radiomics Phantom CT Scans
DOI: 10.7937/K9/TCIA.2017.zuzrml5b | Data Citation Required | Image Collection
Location | Species | Subjects | Data Types | Cancer Types | Size | Status | Updated | |
---|---|---|---|---|---|---|---|---|
Lung Phantom | Human | 17 | RTSTRUCT, CT | Phantom | Image Analyses | Public, Complete | 2017/07/28 |
Summary
This collection consists of 17 CT scans of the Credence Cartridge Radiomics (CCR) phantom, which was designed for use in studies of texture feature robustness. The scans were acquired at four medical centers using each center’s chest protocol and were taken using GE (7 scans), Philips (5 scans), Siemens (2 scans), and Toshiba (3 scans) scanners. The CCR phantom has 10 cartridges, each with a unique texture, Fig 1. The first four cartridges are 3D printed ABS plastic with 20%, 30%, 40%, and 50% honeycomb fill, and they provide regular, periodic textures. The next three cartridges provide natural textures: sycamore wood, cork, and extra dense cork. A cartridges of shredded rubber particles provides textures similar to those of non-small cell lung cancer. The ninth cartridge is solid, homogenous acrylic and provides a minimal texture control. Finally, the tenth cartridge is 3D printed plaster with the highest electron density (400 – 600 HU) and is intended to be more similar to bone. In addition to the DICOM images for the 17 scans, this collection also contains two sets of contours as DICOM RT structure files. The first set provides 8x8x2 cm3 contours for each cartridge in each scan. The second set provides 16 adjacent 2x2x2 cm3 contours for each cartridge in each scan. Researchers studying radiomics will be able to evaluate features for robustness across a variety of scanners. Features can be calculated using the researchers own software or third party software such as IBEX (imaging biomarker explorer). Related publications: Mackin, D., Fave, X., Zhang, L., Fried, D., Yang, J., Taylor, B., Rodriguez-Rivera, E., Dodge, C., Jones, A. K., & Court, L. (2015). Measuring Computed Tomography Scanner Variability of Radiomics Features. In Investigative Radiology (Vol. 50, Issue 11, pp. 757–765). Ovid Technologies (Wolters Kluwer Health). https://doi.org/10.1097/rli.0000000000000180 The following paper was generated on different imaging modalities but the same phantom, this is a related but independent paper with a different set of authors: Fave, X., Mackin, D., Yang, J., Zhang, J., Fried, D., Balter, P., Followill, D., Gomez, D., Kyle Jones, A., Stingo, F., Fontenot, J., & Court, L. (2015). Can radiomics features be reproducibly measured from CBCT images for patients with non‐small cell lung cancer? In Medical Physics (Vol. 42, Issue 12, pp. 6784–6797). Wiley. https://doi.org/10.1118/1.4934826
Data Access
Version 1: Updated 2017/07/28
Title | Data Type | Format | Access Points | Subjects | License | |||
---|---|---|---|---|---|---|---|---|
Images and Radiation Therapy Structures | RTSTRUCT, CT | DICOM | Download requires NBIA Data Retriever |
17 | 17 | 51 | 2,672 | CC BY 3.0 |
Citations & Data Usage Policy
Data Citation Required: Users must abide by the TCIA Data Usage Policy and Restrictions. Attribution must include the following citation, including the Digital Object Identifier:
Data Citation |
|
Mackin, D., Ray, X., Zhang, L., Fried, D., Yang, J., Taylor, B., Rodriguez-Rivera, E., Dodge, C., Jones, A., & Court, L. (2017). Data From Credence Cartridge Radiomics Phantom CT Scans (CC-Radiomics-Phantom) [Data set]. The Cancer Imaging Archive. https://doi.org/10.7937/K9/TCIA.2017.zuzrml5b |
Detailed Description
Supporting Documentation and Metadata
Acquisition parameters for the phantom scans in this Collection:
Scan |
Manufacturer |
Model |
Kernel |
Type |
Slice Thickness (mm) |
Pixel (mm) |
Spiral Pitch Factor |
kVp |
Effective mAs |
CTDIvol (mGy) |
CCR1-GE1 |
GE |
Discovery CT750 HD |
standard |
helical |
2.5 |
0.49 |
0.98 |
120 |
81 |
6.19 |
CCR1-GE2 |
GE |
Discovery CT750 HD |
standard |
axial |
2.5 |
0.70 |
1.00 |
120 |
300 |
|
CCR1-GE3 |
GE |
Discovery CT750 HD |
standard |
helical |
2.5 |
0.78 |
0.98 |
120 |
122 |
9.3 |
CCR1-GE4 |
GE |
Discovery ST |
standard |
helical |
2.5 |
0.98 |
1.35 |
120 |
143 |
16.3 |
CCR1-GE5 |
GE |
LightSpeed RT |
standard |
helical |
2.5 |
0.98 |
0.75 |
120 |
1102 |
53.6 |
CCR1-GE6 |
GE |
LightSpeed RT16 |
standard |
helical |
2.5 |
0.98 |
0.94 |
120 |
367 |
18.8 |
CCR1-GE7 |
GE |
LightSpeed VCT |
standard |
helical |
2.5 |
0.74 |
0.98 |
120 |
82 |
|
CCR1-P1 |
Philips |
Brilliance Big Bore |
B |
helical |
3.0 |
0.98 |
0.94 |
120 |
320 |
17.8 |
CCR1-P2 |
Philips |
Brilliance Big Bore |
C |
helical |
3.0 |
0.98 |
0.94 |
120 |
369 |
15.8 |
CCR1-P3 |
Philips |
Brilliance Big Bore |
B |
helical |
3.0 |
1.04 |
0.81 |
120 |
320 |
19.9 |
CCR1-P4 |
Philips |
Brilliance Big Bore |
B |
helical |
3.0 |
1.04 |
0.81 |
120 |
369 |
19.9 |
CCR1-P5 |
Philips |
Brilliance 64 |
B |
helical |
3.0 |
0.98 |
0.67 |
120 |
372 |
16.4 |
CCR1-S1 |
Siemens |
Sensation Open |
B31s |
axial |
3.0 |
0.54 |
1.00 |
120 |
26 – 70 |
1.5 |
CCR1-S2 |
Siemens |
SOMATOM Definition Flash |
[‘I70f’, ‘2’] |
helical |
2.0 |
0.52 |
0.60 |
120 |
17 – 28 |
|
CCR1-T1 |
Toshiba |
Aquilion |
FC18 |
helical |
3.0 |
0.63 |
1.11 |
120 |
135 |
4.0 |
CCR1-T2 |
Toshiba |
Aquilion |
FC18 |
helical |
3.0 |
0.63 |
1.11 |
120 |
135 |
3.8 |
CCR1-T3 |
Toshiba |
Aquilion ONE |
FC18 |
helical |
3.0 |
0.98 |
0.99 |
120 |
151 |
13.5 |
Acknowledgements
This data set was provided to TCIA by Authors: Mackin, Dennis; Fave, Xenia; Zhang, Lifei; Fried, David; Yang, Jinzhong; Taylor, Brian; Rodriguez-Rivera, Edgardo; Dodge, Cristina; Jones, Aaron Kyle; and Court, Laurence.
Related Publications
Research Community Publications
The Collection authors suggest the below will give context to this dataset:
- Mackin, D., Fave, X., Zhang, L., Fried, D., Yang, J., Taylor, B., Rodriguez-Rivera, E., Dodge, C., Jones, A. K., & Court, L. (2015). Measuring Computed Tomography Scanner Variability of Radiomics Features. In Investigative Radiology (Vol. 50, Issue 11, pp. 757–765). Ovid Technologies (Wolters Kluwer Health). https://doi.org/10.1097/rli.0000000000000180
- The following paper was generated on different imaging modalities but the same phantom, this is a related but independent paper with a different set of authors:
Fave, X., Mackin, D., Yang, J., Zhang, J., Fried, D., Balter, P., Followill, D., Gomez, D., Kyle Jones, A., Stingo, F., Fontenot, J., & Court, L. (2015). Can radiomics features be reproducibly measured from CBCT images for patients with non-small cell lung cancer? In Medical Physics (Vol. 42, Issue 12, pp. 6784–6797). Wiley. https://doi.org/10.1118/1.4934826
TCIA maintains a list of publications which leverage our data. If you have a manuscript you’d like to add please contact TCIA’s Helpdesk.
- Gu, J., Li, B., Shu, H., Zhu, J., Qiu, Q., & Bai, T. (2022). Development and verification of radiomics framework for computed tomography image segmentation. Medical Physics, 1-32. doi:10.1002/mp.15904.
- Ibrahim, A., Barufaldi, B., Refaee, T., Silva Filho, T. M., Acciavatti, R. J., Salahuddin, Z., . . . Lambin, P. (2022). MaasPenn radiomics reproducibility score: A novel quantitative measure for evaluating the reproducibility of CT-based handcrafted radiomic features. Cancers, 14(7), 1599. doi:10.3390/cancers14071599.
- Ibrahim, A., Refaee, T., Leijenaar, R. T., Primakov, S., Hustinx, R., Mottaghy, F. M., . . . Lambin, P. (2021). The application of a workflow integrating the variable reproducibility and harmonizability of radiomic features on a phantom dataset. PLoS One, 16(5), e0251147. doi:10.1371/journal.pone.0251147.
- Kalendralis, P. (2022). Artificial intelligence applications in radiotherapy: The role of the FAIR data principles. (Ph.D. Dissertation). Maastricht University ,The Netherlands, https://doi.org/10.26481/dis.20221010pk
- Refaee, T., Salahuddin, Z., Widaatalla, Y., Primakov, S., Woodruff, H. C., Hustinx, R., . . . Lambin, P. (2022). CT Reconstruction Kernels and the Effect of Pre- and Post-Processing on the Reproducibility of Handcrafted Radiomic Features. J Pers Med, 12(4). doi:10.3390/jpm12040553.