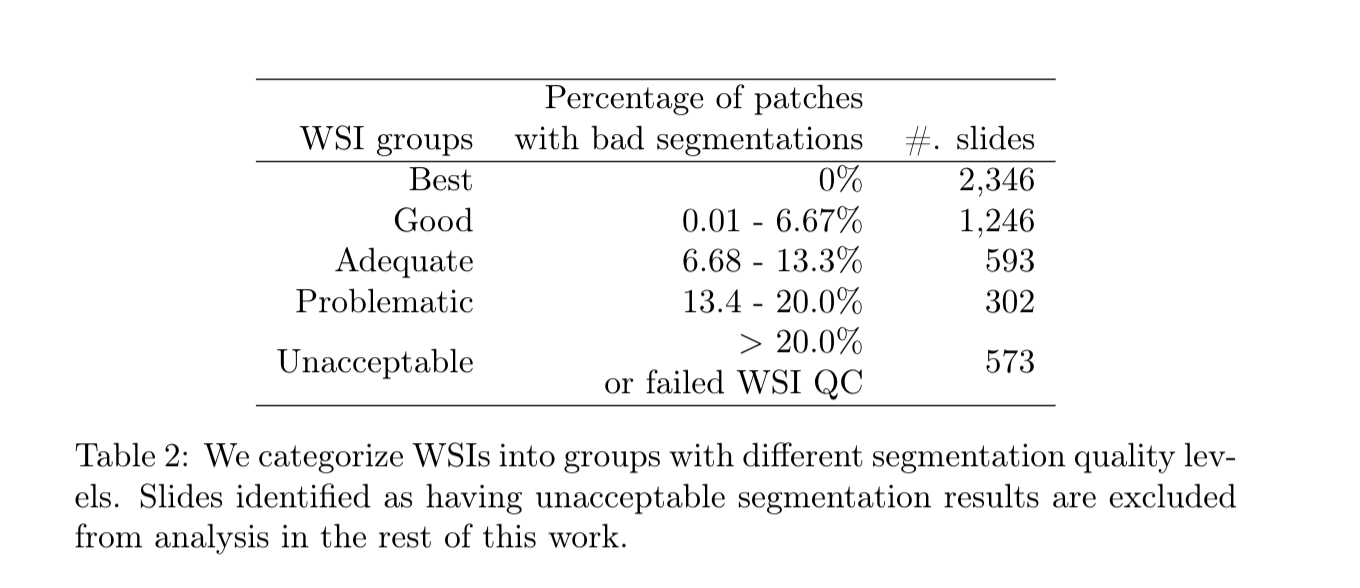
Pan-Cancer-Nuclei-Seg | Dataset of Segmented Nuclei in Hematoxylin and Eosin Stained Histopathology Images
DOI: 10.7937/TCIA.2019.4A4DKP9U | Data Citation Required | 1.1k Views | 2 Citations | Analysis Result
Location | Subjects | Size | Updated | |||
---|---|---|---|---|---|---|
Bladder Urothelial Carcinoma, Breast Invasive Carcinoma, Cervical Squamous Cell Carcinoma, Endocervical Adenocarcinoma, Glioblastoma Multiforme, Lung Adenocarcinoma, Lung Squamous Cell Carcinoma, Pancreatic Adenocarcinoma, Prostate Adenocarcinoma, Skin Cutaneous Melanoma, Uterine Corpus Endometrial Carcinoma, Colon adenocarcinoma, Rectal Adenocarcinoma, Stomach Adenocarcinoma, Uveal Melanoma | Bladder, Brain, Breast, Colon, Eye, Lung, Pancreas, Prostate, Rectum, Stomach, Uterus, Endometrium | 5,204 | Nuclei segmentations | 2020/02/08 |
Summary
Detection, segmentation and classification of nuclei are fundamental analysis operations in digital pathology. Existing state-of-the-art approaches demand extensive amounts of supervised training data from pathologists and may still perform poorly in images from unseen tissue types. We propose an unsupervised approach for histopathology image segmentation that synthesizes heterogeneous sets of training image patches, of every tissue type. Although our synthetic patches are not always of high quality, we harness the motley crew of generated samples through a generally applicable importance sampling method. This proposed approach, for the first time, re-weighs the training loss over synthetic data so that the ideal (unbiased) generalization loss over the true data distribution is minimized. This enables us to use a random polygon generator to synthesize approximate cellular structures (i.e., nuclear masks) for which no real examples are given in many tissue types, and hence, GAN-based methods are not suited. In addition, we propose a hybrid synthesis pipeline that utilizes textures in real histopathology patches and GAN models, to tackle heterogeneity in tissue textures. Compared with existing state-of-the-art supervised models, our approach generalizes significantly better on cancer types without training data. Even in cancer types with training data, our approach achieves the same performance without supervision cost. In this dataset we release code and nucleus segmentations in whole slide tissue images with quality control results for Whole Slide Images (WSI) in The Cancer Genome Atlas (TCGA) repository from 5,204 subjects (6,142 slide images). Within this total, there are two subsets of data: (1) automatic nucleus segmentation data of 5,060 whole slide tissue images of 10 cancer types, with quality control results, and (2) manual nucleus segmentation data of 1,356 image patches from the same 10 cancer types plus additional 4 cancer types. BLCA Bladder urothelial carcinoma Note that you can also download segmentation data of following 4 cancer types, although they are not officially verified. COAD Colon adenocarcinoma5,060 Whole Slide Images (WSIs) are from the following 10 cancer types:
BRCA Breast invasive carcinoma
CESC Cervical squamous cell carcinoma and endocervical adenocarcinoma
GBM Glioblastoma Multiforme
LUAD Lung adenocarcinoma
LUSC Lung squamous cell carcinoma
PAAD Pancreatic adenocarcinoma
PRAD Prostate adenocarcinoma
SKCM Skin Cutaneous Melanoma
UCEC Uterine Corpus Endometrial Carcinoma
READ Rectal adenocarcinoma
STAD Stomach adenocarcinoma
UVM Uveal Melanoma
Data Access
Version 1: Updated 2020/02/08
Title | Data Type | Format | Access Points | Subjects | License | Metadata | |||
---|---|---|---|---|---|---|---|---|---|
Pathology Tissue Slide Segmentation Results | Pathology Detail | SVS | Download requires IBM-Aspera-Connect plugin |
5,204 | 6,142 | CC BY 3.0 | — | ||
List of verified histopathology slides from subset containing 10 cancer types (5,060 images) | Other | TXT | 4,187 | CC BY 3.0 | — | ||||
Whole slide image-level quality control results | Measurement | TXT | CC BY 3.0 | — | |||||
Segmentation region checking results | Other | TXT | CC BY 3.0 | — | |||||
Readme | Other | DOCX | CC BY 3.0 | — | |||||
crosswalk between patch filenames and TCGA case identifiers | Other | TXT | CC BY 3.0 | — |
Additional Resources For This Dataset
Additional information about
- Additional visual segmentation data can be found on PathDB
- Manual nucleus segmentation data of 1,365 patches: These 1,365 patches are randomly extracted from all 14 cancer types mentioned above. This data contains original H&E stained histopathology image patches, and instance-level segmentation masks. the process is in the readme.docx file and
- a crosswalk between patch filenames and TCGA case identifiers are within Pan-Cancer-Nuclei-Seg_1365patches_to_TCGA-ID_readme.txt file.
Citations & Data Usage Policy
Data Citation Required: Users must abide by the TCIA Data Usage Policy and Restrictions. Attribution must include the following citation, including the Digital Object Identifier:
Data Citation |
|
Hou, L., Gupta, R., Van Arnam, J. S., Zhang, Y., Sivalenka, K., Samaras, D., Kurc, T., & Saltz, J. H. (2019). Dataset of Segmented Nuclei in Hematoxylin and Eosin Stained Histopathology Images of 10 Cancer Types [Data set]. The Cancer Imaging Archive. https://doi.org/10.7937/TCIA.2019.4A4DKP9U |
Detailed Description
Additional visual segmentation data can be found on PathDB
Manual nucleus segmentation data of 1,365 patches
These 1,365 patches are randomly extracted from all 14 cancer types mentioned above. This data contains original H&E stained histopathology image patches, and instance-level segmentation masks. Additional information about the process is in the readme.docx file and a crosswalk between patch filenames and TCGA case identifiers are within Pan-Cancer-Nuclei-Seg_1365patches_to_TCGA-ID_readme.txt file.
Related Publications
Publications by the Dataset Authors
The authors recommended the following as the best source of additional information about this dataset:
Publication Citation |
|
Hou, L., Agarwal, A., Samaras, D., Kurc, T. M., Gupta, R. R., & Saltz, J. H. (2019, June). Robust Histopathology Image Analysis: To Label or to Synthesize? 2019 IEEE/CVF Conference on Computer Vision and Pattern Recognition (CVPR). In Proceedings of the IEEE Conference on Computer Vision and Pattern Recognition , pp. 8533-8542. https://doi.org/10.1109/cvpr.2019.00873 Open Access Here |
Research Community Publications
TCIA maintains a list of publications that leveraged this dataset. If you have a manuscript you’d like to add please contact TCIA’s Helpdesk.